%load_ext autoreload
%autoreload 2
import os
os.environ["THEANO_FLAGS"] = "floatX=float64"
import logging
import arviz
import matplotlib.pyplot as plt
import numpy as np
import pymc3 as pm
import theano
import theano.tensor as tt
from scipy.integrate import odeint
# this notebook show DEBUG log messages
logging.getLogger("pymc3").setLevel(logging.DEBUG)
import IPython.display
pymc3.ode: Shapes and benchmarking#
Demo Scenario: Simple enzymatic reaction#
The model has two ODEs with 3 parameters in total.
In our generated data, we’ll observe S
and P
at different times to demonstrate how to slice in such cases.
# For reproducibility
np.random.seed(23489)
class Chem:
@staticmethod
def reaction(y, t, p):
S, P = y[0], y[1]
vmax, K_S = p[0], p[1]
dPdt = vmax * (S / K_S + S)
dSdt = -dPdt
return [
dSdt,
dPdt,
]
# Times for observation
times = np.arange(0, 10, 0.5)
red = np.arange(5, len(times))
blue = np.arange(12)
x_obs_1 = times[red]
x_obs_2 = times[blue]
y0_true = (10, 2)
theta_true = vmax, K_S = (0.5, 2)
sigma = 0.2
y_obs = odeint(Chem.reaction, t=times, y0=y0_true, args=(theta_true,))
y_obs_1 = np.random.normal(y_obs[red, 0], sigma)
y_obs_2 = np.random.normal(y_obs[blue, 1], sigma)
fig, ax = plt.subplots(dpi=120)
plt.plot(x_obs_1, y_obs_1, label="S", linestyle="dashed", marker="o", color="red")
plt.plot(x_obs_2, y_obs_2, label="P", linestyle="dashed", marker="o", color="blue")
plt.legend()
plt.xlabel("Time (Seconds)")
plt.ylabel(r"$y(t)$")
plt.show()
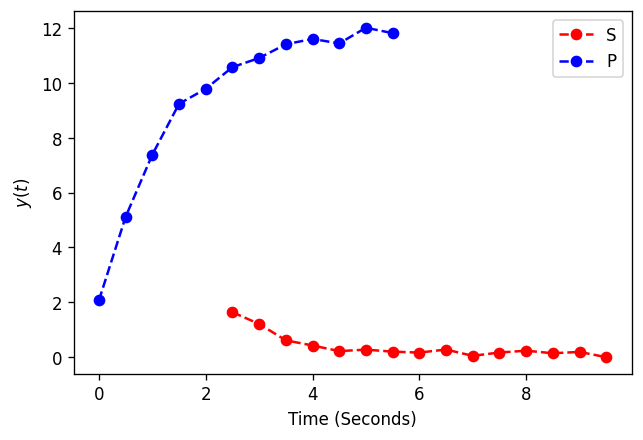
# To demonstrate that test-value computation works, but also for debugging
theano.config.compute_test_value = "raise"
theano.config.exception_verbosity = "high"
theano.config.traceback.limit = 100
def get_model():
with pm.Model() as pmodel:
sigma = pm.HalfCauchy("sigma", 1)
vmax = pm.Lognormal("vmax", 0, 1)
K_S = pm.Lognormal("K_S", 0, 1)
s0 = pm.Normal("red_0", mu=10, sigma=2)
y_hat = pm.ode.DifferentialEquation(
func=Chem.reaction, times=times, n_states=len(y0_true), n_theta=len(theta_true)
)(y0=[s0, y0_true[1]], theta=[vmax, K_S], return_sens=False)
red_hat = y_hpt.T[0][red]
blue_hat = y_hpt.T[1][blue]
Y_red = pm.Normal("Y_red", mu=red_hat, sigma=sigma, observed=y_obs_1)
Y_blue = pm.Normal("Y_blue", mu=blue_hat, sigma=sigma, observed=y_obs_2)
return pmodel
def make_benchmark():
pmodel = get_model()
# select input variables & test values
t_inputs = pmodel.cont_vars
# apply transformations as required
test_inputs = (np.log(0.2), np.log(0.5), np.log(1.9), 10)
# create a test function for evaluating the logp value
print("Compiling f_logpt")
f_logpt = theano.function(
inputs=t_inputs,
outputs=[pmodel.logpt],
# with float32, allow downcast because the forward integration is always float64
allow_input_downcast=(theano.config.floatX == "float32"),
)
print(f"Test logpt:")
print(f_logpt(*test_inputs))
# and another test function for evaluating the gradient
print("Compiling f_logpt")
f_grad = theano.function(
inputs=t_inputs,
outputs=tt.grad(pmodel.logpt, t_inputs),
# with float32, allow downcast because the forward integration is always float64
allow_input_downcast=(theano.config.floatX == "float32"),
)
print(f"Test gradient:")
print(f_grad(*test_inputs))
# make a benchmarking function that uses random inputs
# - to avoid cheating by caching
# - to get a more realistic distribution over simulation times
def bm():
f_grad(
np.log(np.random.uniform(0.1, 0.2)),
np.log(np.random.uniform(0.4, 0.6)),
np.log(np.random.uniform(1.9, 2.1)),
np.random.uniform(9, 11),
)
return pmodel, bm
model, benchmark = make_benchmark()
print("\nPerformance:")
%timeit benchmark()
Applied log-transform to sigma and added transformed sigma_log__ to model.
Applied log-transform to vmax and added transformed vmax_log__ to model.
Applied log-transform to K_S and added transformed K_S_log__ to model.
make_node for inputs -5657601466326543627
Compiling f_logpt
Test logpt:
[array(5.73420592)]
Compiling f_logpt
grad w.r.t. inputs -5657601466326543627
Test gradient:
[array(-12.25120609), array(26.04782273), array(-9.38484546), array(11.26454126)]
Performance:
23.7 ms ± 429 µs per loop (mean ± std. dev. of 7 runs, 10 loops each)
Inspecting the computation graphs#
If you zoom in to the large DifferentialEquation
ellipse in the top, you can follow the blue arrows downwards to see that the gradient is directly passed from the original DifferentialEquation Op node.
theano.printing.pydotprint(tt.grad(model.logpt, model.vmax), "ODE_API_shapes_and_benchmarking.png")
IPython.display.Image("ODE_API_shapes_and_benchmarking.png")
grad w.r.t. inputs -5657601466326543627
The output file is available at ODE_API_shapes_and_benchmarking.png

With the cell below, you can visualize the computation graph interactively. (The HTML file is saved next to this notebook.)
If you need to install graphviz/pydot
, you can use these commands:
conda install -c conda-forge python-graphviz
pip install pydot
from theano import d3viz
d3viz.d3viz(model.logpt, "ODE_API_shapes_and_benchmarking.html")
%load_ext watermark
%watermark -n -u -v -iv -w
numpy 1.18.5
pymc3 3.9.0
theano 1.0.4
logging 0.5.1.2
IPython 7.15.0
arviz 0.8.3
last updated: Sat Jun 13 2020
CPython 3.7.7
IPython 7.15.0
watermark 2.0.2